In this episode of the Unicorny podcast, Dom Hawes talk to Steven Millman, Global Head of Research and Data Science at Dynata.
The conversation focuses on the practical applications of AI in marketing and market research, including customer targeting, personalisation, and campaign optimisation.
Steven and Dom discuss recommendation systems, AI as a service vendors, and the future trends of AI in various industries. They touch on the importance of ethics, team dynamics, and the environmental impact of AI.
If you're interested in learning about the benefits and challenges of AI, as well as its potential impact on society, this episode is a must-listen. So, grab your earbuds and get ready to learn!
This episode is sponsored by Selbey Anderson.
About Steven Millman
Executive, Award-Winning Researcher/Data Scientist, Innovator, Inventor & Coffee Snob. Throughout my career I have had a focus on quantitative/statistical analysis, survey design, research design, AI/ML, and other applied research techniques. I am presently serving as Global Head of Research and Data Science at Dynata, the world's sixth largest market research company where I lead a team of over 100 researchers and data scientists. I am a frequent speaker and author, multiple Ogilvy award winner, patent holder, and recipient of the prestigious Chairman's Prize from the Publishing & Data Research Forum. Steven serves as a member of the Board of Trustees for the Advertising Research Foundation, the ARF.
Links
Full show notes: Unicorny.co.uk
LinkedIn: Steven Millman | Dom Hawes
Websites: Dynata |
Unicorny related episodes:
A/B seeing ya! Is AI the end of split testing? (18:13)
Everything, Everywhere, All at Once
Marketing Trek related episodes:
Data Ethics in Marketing with Steven Millman
Other items referenced in this episode:
DriverTagsTM (18:42)
DevOps (28:17)
Gene Kim: The Unicorn Project (28:17)
State of Digital (40:24)
Unicorny Blogs (40:24)
Timestamped summary of this episode
00:00:03 - Introduction
The host introduces the guest, Steven Millman, as the global head of research and Data science at Dynata. They discuss their previous conversation on large language models and their plan to explore the topic of walled gardens in this episode.
00:01:23 - Cutting Edge Applications
Steven explains that at Dynata, their focus is on helping people make data-driven decisions. They work on data quality, measuring the effectiveness of advertising, and developing data representation systems. They constantly evaluate new technologies and incorporate those that are practical and effective.
00:04:13 - What is a Walled Garden?
The concept of a walled garden originated from social media platforms like Facebook. In the context of generative AI, a walled garden refers to limiting the information fed into large language models. This can be done by narrowing down the data sources or focusing the model on specific use cases. It also involves locking down the model to ensure consistency and avoid potential risks.
00:08:12 - Benefits of Walled Gardens
Walled gardens offer several advantages for businesses. They allow for the inclusion of specific intellectual property, ensuring consistent and secure results. By keeping the data within the organization, confidentiality is maintained, and the risk of sharing sensitive information with competitors is minimized. Building a walled garden may require external vendors with expertise in this area.
00:10:56 - Leveraging AI Applications
The conversation shifts to the availability of AI applications built by vendors.
00:13:51 - The Importance of Data Ownership and Walled Gardens
The discussion begins with the importance of data ownership and the need to keep data private. The concept of walled gardens is introduced as a way to ensure data remains under control. Building, developing, or buying applications that prioritize data privacy is discussed as a solution.
00:14:46 - Practical Applications of AI in Marketing
AI is being used effectively in marketing for customer targeting, personalization, and campaign optimization. Recommendation systems, such as those used by streaming services like Netflix and Spotify, are mentioned as examples.
00:18:39 - AI for Optimizing Customer Revenue Conversions
Dom mentions a past episode- “A/B seeing ya! Is AI the end of split testing?” with Julian Thorne from Sub(x), a company offering AI as a service to optimize customer revenue conversions. Their approach goes beyond simple recommendation algorithms and incorporates sophisticated parameters for personalized messaging and ad performance.
00:19:45 - DriverTagsTM and Emotional Response
DriverTagsTM are discussed as content analytics markers that help understand and shape viewing behaviour in television and films. They can also be used to improve advertising effectiveness by finding the right audience and context. DriverTagsTM offer a way to break out of the limitations of traditional recommendation systems.
00:23:02 - AI in Predictive Analytics and Marketing Automation
Predictive analytics using AI, such as decision trees and neural networks, is mentioned as a valuable tool for forecasting trends and making predictions. Marketing automation platforms
00:27:36 - Privacy and Ethical Considerations
Considerations for privacy and ethics should be integrated throughout the AI development process. As the process evolves, additional ethical concerns may arise. Data privacy is a clear consideration, but other ethical issues may emerge during the development of AI tools and language models.
00:28:05 - DevOps Approach
The development of cross-functional teams in AI development is similar to the DevOps approach. Building teams that integrate various expertise and functions is crucial for successful AI development. The book "The Unicorn Project" by Gene Kim provides insights on building such teams.
00:28:49 - Celebrating Progress
Celebrating milestones and successes throughout the AI development process is important to keep the team engaged and motivated. Recognizing achievements and sharing progress with executive leadership helps maintain excitement and momentum.
00:29:23 - Future Trends
The future of AI development is likely to involve training models in multimodal media, incorporating audio, video, and images. The concept of a "co-pilot" AI, similar to the emergency medical hologram from Star Trek, is expected to become more prevalent in various industries. Another important consideration is the need for more computationally efficient models to reduce the environmental impact of AI.
00:33:15 - Regulation and Transparency
Regulation around AI is a topic of discussion globally. The European Union is developing laws focused on concerning aspects such as facial recognition and the spread of fake news. Transparency in AI models and understanding their decision-making processes will likely be areas
00:36:15 – Further thinking
Host, Dom Hawes, discusses some of his thoughts on this week’s episode. He emphasizes the importance of distinguishing between different architectures and methodologies that support these systems. He also highlights the cross-functional nature of teams necessary to commercialise AI in your business."
This podcast uses the following third-party services for analysis:
Chartable - https://chartable.com/privacy
Please note: This transcript has been created using fireflies.ai – a transcription service. It has not been edited by a human and therefore may contain mistakes.
00:03
Dom Hawes
Today, I am delighted to welcome an old friend back to the studio in Steven Millman, global head of research and Data science at Dynata. And we're going to pick up where we left off in the recent episode called AI. Everything everywhere all at once Now, Steven lives and works in the US of A, and when he lands in London, he swings by our you, so I can add chunks of his immense knowledge to our body of learning. I'm always pleased to see Steven because I always learn so much and he's such a nice guy to boot. Last time I met Steven, we talked about large language models with specific reference to the open source models, in particular Chat GPT. At the end of the show, Steven mentioned walled gardens and I said then that I wanted to pick that up on a future show, and this is indeed that show.
00:54
Dom Hawes
So in a minute, we're going to explore what a walled garden is in the context of large language models. But first, I wanted to find out a little more about the work Steven's doing at Dynata and what cutting edge looks like when you're right on the front line of data AI, marketing and research. Hello, Steven, and welcome back to Unicorny. I think we've done four pods together now, but I don't think I've ever asked you what you're doing in the day job. I think we did a little bit in marketing, trek, maybe, when we talked about the work you were doing around expanding cohorts, using Facebook, but I think that's as much as we've done. So, in your business today, what's leading edge? What are the applications people are using, I mean, specifically AI for and what's most popular? I know it's a big question and it is big because yours is a big company.
01:46
Steven Millman
It's a big company. So at Dynata, if you boil it down to the very basic, our job is to help someone with a question that they need to answer, a decision they need to make, to help them make that be a data driven decision. Anything we do falls into that category. And so world's largest online panel. So we're a massive first party data provider. With respect to this question about what's sort of cutting edge in our space, a couple of areas. One is our application to data quality, bringing these really cutting edge technologies to rooting out fraud, to helping to understand respondent, engagement, people taking surveys, whether they're engaged, so it's a real person or not a real person. And then is that person providing data that we think we can trust? Or is it are they not? And there's a lot of very advanced algorithms being placed into that.
02:36
Steven Millman
There's machine learning as part of that to really understand things. If you think about our process, it's called Quality Score, which is about 196 different checks. It's not like we say, well, if you fail five of them, you're done. So there's this machine learning on the background that looks at the intercorrelations between all of these things and it gives you a really good idea on a person by person basis as they're taking the survey. Before they get to the end, it's going to tell us that this is probably valid or probably invalid. We do a lot of work on measuring the effectiveness of advertising, so we're doing some really interesting things on the creative evaluation front. Is the ad likely to work based on a massive amount of prior data? Is your ad campaign in field working? It's actually very difficult to stitch all that information together because you can't measure every different media channel the same way.
03:29
Steven Millman
So I got to measure TV one way, or actually maybe two or three ways. I measure digital in one way, but I can't measure programmatic the way I measure Facebook, it's that kind of thing. We also have data representation systems that are quite good, that help people understand them. And every day there's some new interesting technology and smart people on the product team, smart people on the technology team, smart people on my team, we investigate them, we separate out the stuff that is snake oil from the stuff that is real and practical and is a today technology and we build them in.
04:06
Dom Hawes
Well, that's great context.
04:08
Dom Hawes
Thank you.
04:09
Dom Hawes
Now, walled gardens, before exploring them, I think we'd better define them. So in the context of tech, what is a walled garden?
04:17
Steven Millman
The term walled garden in this context really started to arise with the Facebooks of the world and the social media, where they were taking what was intended to be this large democratized information and communication system, the internet, the world Wide web, and began to coordinate off and make it so that there were these members only groups. AOL, I think was one of the really early walled gardens. And so this term is now being applied to generative AI in two ways. The first is that they are constraining what information goes into these foundational models. The foundational model being the model that drives the large language model and gives you the answers you want. So if you look at Chat GPT is this massive 175,000,000,000 pieces of information go into, it draws from everywhere on the internet, all kinds of information and it will do all manner of things that you ask it to do.
05:11
Steven Millman
But if you are, for example, looking for a large language model that is going to help you with diagnosing a patient, it doesn't need to do so in the voice of E. Cummings or Robert Frost. It's not an important thing for it to know. And the more information it has to draw on in some ways, the more likely it is that it could hallucinate and come up with answers that are simply unresponsive or flat wrong. So one way you think about walled gardens is you limit the amount of data and information that it has access to in the foundational model so that it is less likely to stray. And I'll give you a couple real quick examples. Bert is really this kind of a model where they have a variety of different kinds of smaller, much smaller language models that are really tightly aligned. So there's BioBERT, for example, for biological, there's legal, Bert for legal, so there's those.
06:08
Steven Millman
But then there's also very structured single organization use cases. So if you have complicated products, require a lot of technical support, you might want to train these large language models to help you with a chat bot that are trained on all of your documentation. You might have hundreds of thousands of pages of technical documentation for certain kinds of products or services. And if you train the language model very tightly on those, it is much less likely that your chatbot is going to stray off or do something that, as we talked about last time, these very large models, they do have bias in them. They can have unpleasant information or false information from the internet built into them. They are representations of all the bad things and good things and true things and false things that are in the training set. So if you exclude all of that and only really allow it to focus in on the documents you care about, it's going to be much less likely to present risk to have that be client facing.
07:10
Steven Millman
The second way people think about Walled Gardens is locking down your instance of a large language model. So I might be using a commercially available large language model or an open source large language model, but if I want to build a scaled enterprise solution on top of that, I can't allow the risk that those models might be changed. And you heard in the news recently over the last few months, is Chachi PT getting dumber? Not really getting dumber. They changed the way it was allocating memory and some of the ways that it's approaching how to pull information out. And they were experimenting with different processes like compartmentalizing certain kinds of information so it doesn't have to hit the entire model in order to get information, can hit little subsets. And as a result, it started to do some things really well that it was doing poorly before, but it also started doing some things poorly that it had been doing well before.
08:04
Steven Millman
And some of the things that had been doing poorly were some of the things that researchers were actually testing as a way to see whether or not these models are getting better or worse. So you can't have those models shifting around on you if you're building client delivery on top of it, right? And so Walled Garden is also a way where you can sort of lock down one of these models and not have it be subject to whatever the owners or the producers of these large language models are doing, and that makes it a lot safer. Other things that Walled Gardens do for a company if you make your own large language model platform is, it also allows you to avoid one of the major risks, which is that you are feeding your private information or PII personally identifiable information or personal health information. None of that stuff can go back to the source of the language model because you're not connected to it from.
08:54
Dom Hawes
A commercial point of view. Then wall Gardens, number one, it's a much more effective way of getting your specific intellectual property right into a model that can help then service your clients and your business.
09:05
Steven Millman
Yes.
09:05
Dom Hawes
Number two, it's going to give you a lot more consistent results, which of course, consistency is really important when you're trying to build commercial grade applications like that. You're reducing your security risk because it's only your data. So I mean security in its widest sense. So you're not going to offend upset breach any laws, hopefully, because your data.
09:24
Steven Millman
Set is your data set, ideally, not.
09:26
Dom Hawes
Exactly, and you're not giving your confidential information away to competitors. I think how people go about building a wall garden is probably outside the scope of what we're talking about today or what they might do to do that.
09:38
Steven Millman
I would say that not a lot of organizations have the in house capabilities to do this stuff, but there are now actually an expanding number of vendors who are out there building these for you.
09:48
Dom Hawes
Yeah, I've seen a few of those start to appear. We use three different vendors, for example.
09:53
Dom Hawes
To help produce this show, cap show.
09:54
Dom Hawes
Chat, GPT itself, and Fireflies. And in my day work, I've also started to see applications that are claiming to be able to use large language models to open up your own SharePoint and Azure Knowledge Bases sana comes to mind.
10:08
Dom Hawes
They're a Stockholm based Series B AI.
10:12
Dom Hawes
Powered learning platform, and I'm going to look a little bit more into them.
10:16
Steven Millman
Yeah, and then there's others out there I'm particularly impressed right now by Databricks.
10:21
Dom Hawes
Okay.
10:21
Steven Millman
They've got a whole suite of products where they'll handle both your data architecture and the large language model. They bought Mosaic a little while back.
10:29
Dom Hawes
And this sort of plays to what you were saying last time, that a lot of us are using artificial intelligence or we get the benefit of it through applications that other people are building. So we don't actually need to build our own Data science or AI department because the applications that we're using every day will just have it built in like Salesforce or Office already does.
10:48
Steven Millman
Yeah, exactly. But like those things, you absolutely have to have staff on hand who understand in a great detail how they work. But I do think that as a service, that's definitely the model where most companies are going to go to.
11:01
Dom Hawes
One other thing that just sprung to mind while were talking. Then I was at an event the other night with about 20 other people. They were marketers from different businesses, and the subject of AI came up, and there wasn't a great deal of knowledge in the room, I suppose, which is understandable, including me, obviously. But the distinction between just having data and being able to analyze data and AI, I think probably on many people was slightly lost. And I just wondered whether we might want to get some clarity, because I'm assuming that a lot of people listening to this may not be that data literate either. So in terms of data and data science, I guess that's spotting patterns and correlations and trying to understand causation. Whereas when we're talking about AI, we're talking about learning models that get better without human intervention. Is that fair?
11:54
Steven Millman
I mean, it's all kind of mixed up together now in certain ways. There are versions of artificial intelligence that are very effective at predicting, and there are those that aren't. Large language models are not good at predicting. I was having a conversation with some friends, and you remember when Bill Clinton was running for president, is dating for a lot of folks who are going to be listening to this. And I apologize, but he had this big sign in his campaign that said, it's the economy, stupid. I printed out one that looking for a place to hang it in the office that says it's a language model, stupid. Don't expect language models to be great at math. They're not math models. They're language models. Don't expect them to be good at predicting. They're not. The big difference is rules based, business, rule driven models versus models in AI, where the system is figuring out how to answer the question without being told explicitly how to come to answer.
12:48
Steven Millman
And so the kind of classic example is asking an AI to figure out if a picture has a cat in it. And so you show it 10,000 pictures with no cat in it. Say these don't have any cats. You show it 10,000 other pictures, and you say, these have cats. You don't tell it what a cat is. You don't describe the cat. You just say, there is a cat in these. There's not a cat in those. Figure out how to tell me on the next picture you see whether there's a cat, and it will do it in ways that a human wouldn't do it. It's also very hard to sort of peel out exactly how they figure out how to do that. But some very smart data scientists and AI people have figured out how to extract some of this. And one of the things was that it was looking at the tip of the cat's ear.
13:32
Steven Millman
There's something about the tip, just that very point of the cat's ear, which would be virtually invisible to a human looking at it that it recognized was in pictures with cats that wasn't in pictures without cats, that would never be something we would think about. And so that's really the difference is that I haven't explained to the computer anything about how to solve the problem. All I have said is I have a problem and tell me how to answer it.
13:54
Dom Hawes
Let's move on.
13:54
Dom Hawes
And so I think that's a really good starting point to look at wall gardens and to understand the concept that your data still needs to stay your data, and you don't want to share it with anybody. But of course you're building it to help you do things, which means you've got to build, develop, or buy. Of course we've talked about buying applications that have this built in and then deploy it.
14:15
Dom Hawes
And so I'm interested to move on.
14:17
Dom Hawes
To look in terms of practical applications around marketing and market research. Obviously, what kind of models and algorithms are most useful and kind of what the process for building or buying and deploying these tools might be.
14:30
Steven Millman
First, let me just say we spent a lot of time talking about generative AI in the last podcast, but the answer to this question is not heavily generative AI. There's a lot of different kinds of artificial intelligences out there doing different jobs, and large language models are being now applied to these processes. It's very incipient in most cases. So let me talk about some of the ways that AI are being used and are being very effective in marketing. Primarily, we're talking about customer targeting, we're talking about personalization, and we're talking about campaign optimization. And if you sort of remember the old adage as we do, getting the right ad to the right person at the right time, solving those kinds of problems, give you an idea about a number of different kinds of systems that are commonly in use. So recommendation systems. Anybody who has used a streaming service has been engaging a recommendation system.
15:22
Steven Millman
I had a really interesting conversation with friends of mine on Facebook today, speaking of walled gardens, where someone was complaining that suits, which is on Netflix now, and it's having a huge resurgence. If you go to Netflix and you look for suits, the image it shows you is going to be potentially different depending on who's looking at it. And so a friend of mine said, I can't believe that the picture that they're showing me is this beautiful woman in a bra who had literally 5 seconds of screen time across nine seasons of this show, who's not a main character, sort of blink and you miss it. And I got that. And most of my male friends got that image presented to us when you call that up. But other people got Donna the redhead, and some of the women were getting pictures of some of the men on the show.
16:12
Steven Millman
And personally, I don't know why I was shocked, but I was shocked at how sophisticated that system is that it was working to do that. But beyond that, also the more standard things you'd expect. I watch these kinds of shows. I might very much like these kinds of shows. And it's a way the system can engage you and keep you looking for that next bit of content instead of saying, okay, I think I've exhausted what I want to binge today.
16:36
Dom Hawes
That kind of technology is interesting, but Spotify obviously, is a classic example. And I know they've just started Daylists, which is another version of their discovery engine, and it's meant to be having some intelligence, understanding the type of music you like. But in reality, I think the more you listen, you get that mean reversion effect we talked about last time, where you actually just get more of the same. And my daylists are very rarely anything that I haven't already listened to. So it's not able to make a leap beyond the music that's already in my library.
17:06
Steven Millman
Yeah. And so training matters. The quality of the artificial engine matters, and whether or not the problem that they're trying to solve is being expressed correctly. So if you go back to the cat example, what I really want to know about is whether there's a cat walking around. And I don't tell it that it matters to me that the cat is walking around. I'm not going to get the right answer. I'm just going to get that there's a cat in the picture. It's absolutely true. A lot of recommendation engines will look at the first set of things that you like. It will represent things that are exactly like them and won't be smart enough to then look for intercorlations between that kind of content and other kinds of content. And some of them are, I think. Now, I'm not in the middle of these, but I will say, for example, on Netflix, it will break things out into multiple genres and make recommendations across genres based on commonalities.
18:04
Steven Millman
And I assume that's built into their AI. I don't know for a fact, obviously, because I don't work there, but those are the kinds of advancements you'd expect to see in recommendation systems.
18:13
Dom Hawes
In the episode before last, we spoke to Julian Thorne from Subx. That's a company that's marketing a new generation of AI as a service to optimize customer revenue conversions. And they're targeting publishers now, sort of instead of the if you like that, then you'll like this, which is the next best show for you type algorithm. Vertech offers a next best message for you to optimize conversion based on some pretty sophisticated parameters. It solves some of the problems around.
18:42
Steven Millman
Split testing, and there's other kinds of data that they're using other than just, I watched this show. So a friend of mine named Bill Harvey, really brilliant fellow, helped develop this thing called Driver Tags and Driver Tags. And Bill, if you're listening, I apologize if I get this. Not quite right, but what driver tags are is a series of kind of thoughts and emotions that you can associate with certain kinds of content. And you can also look to see if the emotional drivers of what appears to cause people to want to watch these shows exist in other shows and that would break these genre questions and things like that. I just want a show that's going to make me happy, okay? I want a show that's going to make me feel things in my heart. That's another great way that you can sort of build in more information and get out of the rut that some of these recommendations are definitely in, where it's just saying if any of you remember what a video store was, a video rental store, you would just be looking at the thing next to the thing you were looking at.
19:45
Dom Hawes
So how you doing? It's good stuff, isn't it? I really liked the idea of driver tags, so as soon as we'd finished recording, I went and looked them up and I've added a link to some of the stuff I found on the main show notes at Unicorny.co.uk . Now, it turns out that driver tags are content analytics markers or meta tags that help understand and shape viewing behavior to television and films. And using them, Emmy Award winner Bill Harvey and Bill McKenna turned their attention to advertising effectiveness, and they delivered massive improvements in ad performance through helping to find the people whom an advertisement would really move and to find the contexts that would most amplify an advertisement. I'm going to dig deeper into driver tags because I've got an unscratched itch around measuring emotional response to communication that I think might just get scratched by driver tags.
20:42
Dom Hawes
Anyhow, in the rest of the show, we looked at Walled Gardens and how businesses might be able to reap benefits of training large language models on their own data. Later on, we're going to look at whether future legislation might make investing in.
20:56
Dom Hawes
That kind of technology a little bit risky right now.
20:59
Dom Hawes
But it also seems that at the same time, AI as a service vendors may provide a lower entry price solution for most businesses. And we talked earlier about Sana and Databricks. They seem to be like good examples, certainly at the time that we're recording this. Now, I've been a little bit obsessed.
21:17
Dom Hawes
About this area over the last three.
21:19
Dom Hawes
Years because we've got terabytes and terabytes of data stretching back years, and that data covers highly specific issues in vertical markets like finance, technology, and industrial. And I would dearly love to be able to interrogate that data using natural language searches, and it seems that I may soon be able to do just that. Now, if you've been thinking about this kind of stuff too, I would love.
21:44
Dom Hawes
To talk to you.
21:45
Dom Hawes
So please get in touch with me.
21:46
Dom Hawes
Through the website at Unicorny.co.uk.
21:48
Dom Hawes
Or via my profile on LinkedIn let's.
21:51
Dom Hawes
Now get back to the studio. So right now generative AI interesting, everyone's playing with it, but actually that's not the thing that's driving adoption and usage right now.
22:01
Steven Millman
Yeah, and that's because it's new. It's like being in a fancy hotel, but some rooms are absolute rubbish because they haven't been renovated yet. It's kind of like that. A couple of other areas that this stuff is being used, natural language processing obviously is used everywhere. It's in your phone. Just the ability to take unstructured text and analyze it or to take your voice and turn it into text and so forth. Predictive analytics. There's a lot of AI being used in predictive analytics, not large language models because as the sign says, it's a language model, stupid. But these use historical data to forecast trends and to make predictions about what's going to happen next. And like the cat example I gave you, it does it in ways where we might not as a researcher, I might not see that relationship, I might not see the complexity of the intercorrelations, but the AI can sort that out faster, better, cheaper, and then give you these very reasonable predictions for the future.
23:02
Steven Millman
When you hear about these things, you're usually talking about decision trees and neural networks, that sort of thing.
23:08
Dom Hawes
Is that kind of some of the Einstein stuff that's built into salesforce that'll be doing some of that won't, I guess, looking at patterns and then using that to try and predict future customer behavior?
23:17
Steven Millman
Yeah, I mean, I would presume that they're using neural networks for that or decision tree.
23:21
Dom Hawes
The application of it reminds me of.
23:23
Steven Millman
What we see exactly, economic trend analysis. The ability to do things like take into account seasonality in your sales cycle and predict ahead of time what would be a good outcome and what would be a bad outcome. Because if you're selling candy, you know every February sales are going to go up, but you don't know how much. And so the fact that it went up, everybody's happy. But if it went up less than it should have, you should be unhappy. If it went up more than it should have, you should be very happy. And the dentists might have a different opinion, but that kind of forecasting is absolutely invaluable.
23:59
Dom Hawes
So Steven, what else are you seeing?
24:01
Dom Hawes
What else are people doing with artificial intelligence?
24:03
Steven Millman
Sure. Marketing automation. So using AI driven marketing automation platforms to automate email campaigns. So each email campaign might be a little different for each person receiving it based on what you know about them, what's in your CRM or what's in your lead gen software, et cetera. One more big one I'd like to make sure I talk about are clustering algorithms. So looking for commonalities between groups that may be hard to identify, where, for example, typical clustering techniques like K means or hierarchical might not find it, where there are some folks finding really interesting results and good success applying machine learning to those.
24:43
Dom Hawes
That's a really interesting area. I'm going to go do some research in that and maybe next time we meet we can look at clustering techniques because I think that's really interesting. It's particularly from a marketing point of view as a CMO with media fragmenting all over the place with the Remit, everyone has to do more with less. That kind of technology, I think that would allow you to be more efficient in what you're doing. Could be really important.
25:04
Steven Millman
Yeah, and it's definitely a growing area in my business. We get a lot of requests for custom segmentation.
25:10
Dom Hawes
Let's move on to the more human.
25:11
Dom Hawes
Side of all of this because it's all very well having the technology, knowing how to use it is a different thing. But if you're going to deliver a campaign as an organization that's using this technology, if you're going to embrace it, you're going to have to get collaboration between lots of different types of people who may be working in different types of ways. How are you seeing people effectively build collaborative teams that are embracing all the different skill sets?
25:37
Steven Millman
That's a great question. You've got to make sure that you're fostering collaboration between some folks who don't speak the same language. So between data scientists, between the marketers, between people in finance, between technology. And if you really want to develop effective AI strategies in marketing, you've got to get all these people working together seamlessly. So a couple of really good things to keep in mind when you're doing that, number one is establish clear objectives. Make sure that everybody in all of the teams understands the thing you're trying to accomplish. If you have any member of the team that can't say in one sentence what you're trying to do, you haven't gotten this step right. Make sure the teams are cross functional. It is common for organizations to have completely separate chains for marketing data science, technology. And I'm not saying you shouldn't do that, but if you're going to build these, those folks have to form teams within that.
26:28
Steven Millman
It can't be these blind handoffs from one stoppipe to the other. Get them working together, put them in rooms together, and make sure that you have a team whose membership is clear. Shared Data Access it's a silly thing to say, but a lot of times when people are trying to build these systems, one group will have access to certain materials that another group won't have access to. And it leads to mistakes and failures of understanding data literacy and education. Every member of this team knows something every other member of the team doesn't know. Take the time to educate your peers in different groups. Your data scientists don't know marketing. Your marketers don't know data science. They don't all have to be experts at everything, but they have to understand the basics. Make sure that you take the time to do. That. And then I would say one other thing to keep in mind is, and this is probably true for everything, not just this prototyping and feedback.
27:20
Steven Millman
So don't build the whole thing and then figure it out. Build a prototype, make sure it works. Have that proof of concept, get feedback and build and just keep moving through that loop so that ultimately you don't end up having spent a year and build something that isn't going to work for anybody. I guess one other thing I should probably mention is start with some sense of what are the privacy and ethical considerations that need to be sort of carried throughout the entire process. And it won't be clear necessarily from the beginning. So you may need to evolve that. Things like data privacy are going to be very clear. But as you're building out a tool in AI or with large language models, as this process evolves, you may identify other ethical concerns or they may present themselves in the results. And you just need to continuously keep that in mind and build that into how you're ultimately going to have a system so that system is not going to cause you headaches down the line.
28:17
Dom Hawes
As you were talking to me about that and the development of cross functional teams, that brought to mind the whole DevOps approach, the move from sort of siloed tech development into DevOps. And anyone listening that wants to explore that Gene Kim's book, The Unicorn Project, I think really talks in detail about how to build those kind of cross functional teams. So if you're looking to do some extra research, I thoroughly recommend that book, and I'll link that on the show notes.
28:40
Steven Millman
One thing I didn't mention, and I really feel like I should always mention this, is when you go through these feedback loops and things work, celebrate. Celebrate. People get locked. They feel like they're locked in a basement and they can't show anything. They can't get any feedback. And it's a grind building these things. And when the next component works, take the time to celebrate. Tell executive leadership we've got this great thing. Open a bottle of champagne or get donuts, whatever. Celebrate throughout the process. Keep people engaged. Keep people excited that they're making progress.
29:14
Dom Hawes
Let's now spin onto the future. We like to talk about the future.
29:19
Dom Hawes
On this show kind of trends and.
29:21
Dom Hawes
What you see coming down the line. I think when we originally spoke, I said, what do you see coming in the next five to ten years? But things are moving so quickly that timeline might that might be too long a timeline, but in general terms, what do you see coming down the line?
29:35
Steven Millman
If you'd asked me two years about a thing that I'm trying to build right now, I would have said that's ten or 15 years off. And now it's today technology. So predicting the future. We talked about predictive analytics. I don't know if I can predict the future. But I can tell you, I think a couple of things that I think are going to be consequential. One thing that has become really clear as people are really working on large language models and trying to find ways to use them commercially is that most of what humans do is not text.
30:04
Dom Hawes
Okay.
30:04
Steven Millman
Right. And so I think we're going to start to really move into thinking about how do we train models in multimodal media, so audio like this, video images, and bringing all of that in together and building models that can pull all of that in as part of their training sets. I think another area that we're going to see is I would keep coming back to Star Trek because I feel like we're getting there really fast all of a sudden, but I think we're going to be seeing a lot more industries where everyone is going to have a copilot. Copilot is a common term in the AI world, and particularly in large language models where the models and the technologies aren't doing the job for you, but they are helping you out. And if we have any Star Trek fans and you think about the emergency medical hologram, and I'm not saying we're going to have a hologram walking around and that it's going to be able to do procedures, but you can imagine a doctor walking around with some device that they can ask questions to.
31:07
Steven Millman
Are there any medications, contraindicated for the one I want to give my patient? I think that's going to become ubiquitous in a lot of different jobs. And I think the other one, and I know this is sort of a weird thing to think of when we're talking about AI is sustainability. It's not necessarily commonly understood, but these language models are enormously computationally intensive, and these computers necessary to produce them require excessive cooling and a lot of electricity. If you take a small language model like Burt, which is trained on 110,000,000 parameters, they estimate that consumes about the same amount of energy just to train that model as it would if you took a transcontinental flight if you flew from Los Angeles to London. Now, if you look at one of the big ones, like Chachi PT, so Chachi PT Three, I don't know the numbers for four, but I was reading this earlier.
32:06
Steven Millman
Chachi PT. Three uses 175,000,000,000 billion with a B parameters. And to train that, it's estimated that it consumed 1287 megawatt hours of energy of electricity and generated 552 tons of carbon dioxide. And if you want to put that in context, that's about 123 gas powered cars operating as they do typically in the US. For a whole year. Wow. So I think the other area we're going to go to is how do we build these models in computationally more efficient ways where there's going to be less impact? Because it is weird to think about AI being ecologically dirty, but it is the case right now.
32:53
Dom Hawes
Yeah.
32:53
Dom Hawes
No one really thinks about that at the time. I remember thinking it was a bit of a reach where someone was talking about how email was effectively ecologically unfriendly because you think of it, of course, being electronic on the screen. But of course, as you say, there's a lot of power going on. Now take that to a power of and you've got AI, which I know just a query on Chat GPT is meant to be ten times more resource hungry than a query on Google. What about things like regulation? I know the UN General Assembly is struggling to agree. I know there's been lots of talk about, do we take a six month development moratorium because we're worried about the pace this thing's gathering? I think we touched on regulation maybe very briefly previously. I'm sure the EU is gagging to get out and put some regulation out.
33:33
Dom Hawes
Where do you think we might get in the next few years on that?
33:35
Steven Millman
Yeah, well, I mean, the European Union's law on AI, that one's going to come out. I think I want to say next year, or I think it comes out next year, goes into force next year. I'm not 100% certain. That one is sort of tightly tailored around the most concerning things. Things like the use of facial recognition and the ability to produce fake news, misleading information at scale. It's really focusing on that kind of stuff. The UN is struggling. I know in the United States we're struggling. The challenges I think that we're going to have to face, and I think we're going to start seeing come up in law are going to be things like, can we know transparently what the models are doing? Can we prevent bad things from happening by knowing what's going in? Right now, there's very little transparency and of course, there's very little known about how these models actually are producing what they're producing.
34:26
Steven Millman
Again, it's a strange thing to say, but they talk about these as emergent properties in the same way that they talk about consciousness as an emergent biological process. It does these things and we can repeatedly do these things, but we don't know exactly how they're doing them. And that obviously raises concerns. And so there may be consideration for laws around what kinds of things is it okay to allow to be done this way and what things are not? If you think about this as analogy, if you think about the level of statistical significance, if I want to be confident that an ad is helping me sell orange juice and that it's effective, I can be 90% confident that's okay. But if I want to be confident that a vaccine works and that it doesn't have deadly consequence, I need to be a lot more certain than 90%.
35:13
Steven Millman
And so I think what's going to happen is there's going to be fields where there may be a lot of regulation about how these things are used until they can be transparent and we can understand how they work.
35:22
Dom Hawes
The reason I ask about things like regulation, of course, is if the future direction of regulation is unknown, that is likely to put a dampener on investment like commercial investment in this kind of technology. But I guess the starting point for most people isn't going to be in contentious areas. It's going to be, as you say, as a copilot to deliver better or improved service or lower cost. It's not going to be or it's unlikely to be. Certainly with good actors, it's unlikely to be anything that's going to fulfill a future legislation.
35:51
Steven Millman
I would like to believe that's true.
35:53
Dom Hawes
I'm an optimist. It's my job to be an optimist.
35:55
Steven Millman
I have to say I'm not sure that's true. I think absence of regulation leads to a Wild West approach and there's definitely a lot of emerging players who are cash grab. And I think we are going to see a lot of people taking advantage of the absence of regulation to do bad things that will make them money, just like we have before drugs were regulated, before doctors had to have licenses.
36:21
Dom Hawes
Yes, okay, yeah, I meant good actors being reluctant to invest in case regulation made their investment redundant. But of course, and I did. In fact, someone was commenting one of our previous threads and pointed out fraud GPT to me and other such things that I'm not going to publicize on this podcast.
36:41
Steven Millman
I agree with you. I think good actors will be reluctant to engage certain of these groups, but there's a lot of money being spent by people who don't have great intention. As always.
36:56
Dom Hawes
Well, there you have it. I love speaking to Steven so much so that when we'd finished recording the show, went to the local pub to have a pie and a pint and we carried on talking for at least another hour. And this is such a fascinating area that like Depeche Mode's 1981 hit from the Speak and Spell album, I Just Can't Get Enough. I just can't get enough. So here are some things I'm going to think about more after today's show. Firstly, we need to differentiate between the various architectures and methodologies that underpin these kinds of systems. Steven pointed me to the distinction between rule based models and the more contemporary models that learn without explicit programming. Rule based models function on predefined instructions, so they produce consistent results, but they can be limited. Whereas large language models and the like generate their responses from vast data sets, evolving their outputs as they go based on the information they've been trained on.
37:59
Dom Hawes
What's particularly fascinating and perhaps counterintuitive about these expansive and evolutionary language models is they're non predictive. As Steven said, it's a language model, stupid. So, despite their ability to generate impressively coherent and contextually relevant responses, they explicitly cannot forecast future outcomes or trends. Their strength, therefore, is not in prediction, but in synthesizing, understanding and generating language based on their training. Now, that makes them powerful tools for a range of applications, but completely useless when it comes to thinking about the future. If you want to get into the realms of predictive analytics, you're going to need to use rules based models. Next up, as Steven was speaking to me about the cross functional nature of teams needed to commercialise AI in your business, DevOps came to mind and I mentioned Gene Kim's business novel, The Unicorn Project. I just think that might need to.
39:01
Dom Hawes
Be explained in a little bit more detail.
39:03
Dom Hawes
So DevOps here goes. Right, DevOps first appeared in 2009 when Patrick Dubois found a better way of managing software development and It operations. DevOps emphasizes collaboration, automation and continuous integration. Its core is about breaking down silos between the different departments and skill sets needed to code and bring an app to production. By fostering a culture where teams work in unison towards common goals, it's also about important things like decentralizing and allowing decision making closest to the point of pain. The Unicorn Project's a seminal work on this subject and illustrates how successful the approach can be in transformation. Now, the lines between traditional software development and AI implementation will, in my opinion, begin to blur. The complexities of AI projects from data handling to model training will require a seamless integration of very diverse skill sets. And that is where the principles of DevOps come to the fore.
40:08
Dom Hawes
So by fostering a culture of cross functional collaboration, you can ensure smoother AI project rollouts, faster iterations, and more reliable results. Why the hell does this matter to marketing?
40:21
Dom Hawes
You might be screaming at your earbuds.
40:24
Dom Hawes
Well, because DevOps cousin is revops. It's the same thing applied to customer lifecycle, and it needs a cross functional approach involving sales, marketing, operations and customer success. Now, with AI in the mix too, your cross functional team just got bigger. Study the DevOps transformation and you'll be equipped for your revops transformation too. Well, that is enough for today. Each episode also, by the way, comes with an accompanying blog. You can find that blog either on Unicorny Co UK or on Stateoftdigital.com, and we also sometimes publish a newsletter on LinkedIn. But your best bet is either Unicorny or State of Digital. Now, we're going to link both of those in the show notes. And by the way, the blog to support this episode is about the environmental footprint of AI. I think it's going to surprise you, so be sure to check that out. Thank you for listening today's show.
41:20
Dom Hawes
It's up to you, but most people subscribe and review after listening to two episodes, and you can do that on this Pod platform. You can also register at Unicorny.co.uk. You can leave us a voicemail there too. The buttons on the right hand side of the screen, and we'd love to hear from you. So if you do that, we'd be really grateful. But that's all for now, so thank you and we'll catch you next time.
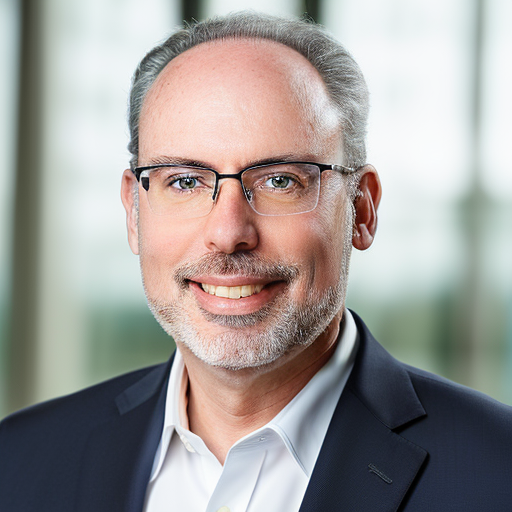
Steven Millman
Global Head of Research & Data Science, Dynata
Executive, Award-Winning Researcher/Data Scientist, Innovator, Inventor & Coffee Snob. Throughout my career I have had a focus on quantitative/statistical analysis, survey design, research design, AI/ML, and other applied research techniques. I am presently serving as Global Head of Research and Data Science at Dynata, the world's sixth largest market research company where I lead a team of over 100 researchers and data scientists. I am a frequent speaker and author, multiple Ogilvy award winner, patent holder, and recipient of the prestigious Chairman's Prize from the Publishing & Data Research Forum. Steven serves as a member of the Board of Trustees for the Advertising Research Foundation, the ARF.